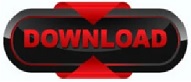
In the Kernel Name field, give your new kernel an appropriate name. The Kernel Properties window now appears, which lets you configure your new kernel. In the window that appears, click Add to create a new kernel profile. The Wolfram System can automate the running of remote kernels.īegin setting up the remote kernel by selecting Evaluation ▶ Kernel Configuration Options: Using a remote kernel to perform your computations is very convenient if your computer has limited hardware resources or is being used for other tasks. The front end is what you see on your screen (graphics, text, and display of your computations), while the kernel takes care of almost everything else, including the vast majority of computations. However, this conclusion should be validated on larger corpora.The Wolfram System can be thought of as two distinct parts, a kernel and a front end. The results obtained on the test set show the relevance of our approach, as the F-measure reaches 88%. Our aim is to show how the combination of similarity measures offers a better semantic access to the document content than only one measure and it improves the performances of the automatic alignment. Three syntactic levels are investigated: Nouns, Nouns-Adjectives, and Nouns-Adjectives-Verbs. We propose a terminology alignment by an SVM classifier trained on a compact, but relevant representation of such definition pair by several similarity measures and the length of de¯nitions. The alignment between a specialized terminology used by the librarians to index concepts and a general vocabulary employed by a neophyte user in order to retrieve documents on Internet, will certainly improve the performances of the information retrieval process. Taken from different dictionaries that could be associated to the same concept although they may have different labels. We propose an original automatic alignment of definitions
However, for the online learning LWPR presents the better method due to its lower computational requirements. The results show that GPR and SVR achieve a superior learning precision and can be applied for real-time control obtaining higher accuracy. Our comparison consists out of the evaluation of learning quality for each regression method using original data from SARCOS robot arm, as well as the robot tracking performance employing learned models.
In this paper, we evaluate these three alternativesįor model learning. With fewer open parameters and potentially higher accuracy. However, while LWPR has had significant impact on learning in robotics,Īlternative nonparametric regression methods such as support vector regression (SVR)Īnd Gaussian processes regression (GPR) offer interesting alternatives Weighted projection regression (LWPR), has been the standard methodĪs it is capable of online, real-time learning for very complex robots. Learning method is suited best for learning dynamics? Traditionally, locally However, a major open question is what nonparametric To using a combination of rigid-body dynamics and handcrafted approximations
In the past, learning has proven a viable alternative Often been limited by the complexities of manually obtaining sufficientlyĪccurate models. In terms of precision or energy efficiency, the practical application has While it is well-known that model can enhance the control performance